1F. Pambudi Widiatmaka, 2Pranyoto,3Didik Dwi Suharso, 4Kundori, 5Riasny dewi Annafril, 6Sukrisno
1,2,3,5Merchant Marine Polytechnic, Singosari Raya No.2A, Kota Semarang, 50242, Indonesia.
4Universitas Maritim AMNI, Sukarno Hatta street No. 180, Pedurungan, Kota Semarang, 50199, Indonesia
6Indonesian College of Tourism Economics, Lamongan Tengah bendan, Kota Semarang, 50233, Indonesia
DOI : https://doi.org/10.47191/ijmra/v7-i11-09Google Scholar Download Pdf
ABSTRACT:
This study examines the relationship between teacher digital competency and technology adoption in maritime vocational education institutions. The research aims to investigate how digital competency influences perceived usefulness, perceived ease of use, behavioral intention, and actual technology use among maritime educators. Using the Technology Acceptance Model (TAM) framework, the study explores the interconnected relationships between these variables in a specialized educational context. Data collection involved an online survey of lecturers and instructors from maritime vocational colleges under the Ministry of Transportation. The study employed structural equation modeling (SEM) using AMOS software for analysis, providing a comprehensive examination of the relationships between variables. Results reveal significant positive relationships between teacher digital competency and technology adoption factors. The findings demonstrate that digital competency strongly influences both perceived usefulness and ease of use of technology, which in turn affects behavioral intention and actual technology implementation. The research contributes to understanding technology adoption in maritime vocational education and provides practical implications for institutions developing digital competency programs. The study suggests that successful technology integration depends on both technical competency development and positive perceptions of technology's utility, supported by adequate institutional infrastructure.
KEYWORDS:Digital Competency, Technology Acceptance Model, Maritime Education, Vocational Training, Educational Technology
REFERENCES1) Abdullah, F., & Ward, R. (2016). Developing a general extended technology acceptance model for E-learning (GETAMEL) by analysing commonly used external factors. Computers in Human Behavior, 56, 238–256. https://doi.org/10.1016/j.chb.2015.11.036
2) Abramson, J., Dawson, M. and Stevens, J. (2015), “An examination of the prior use of e-learning within an extended technology acceptance model and the factors that influence the behavioral intention of users to use m-learning”, SAGE Open, Vol. 5 No. 4, pp. 1-9.
3) Ajzen, I. (1991). The theory of planned behavior. Organizational Behavior and Human Decision Processes, 50(2), 179–211. https://doi.org/10.1016/0749-5978(91)90020-T
4) Ajzen, I., & Fishbein, M. (1975). A Bayesian analysis of attribution processes.
5) Ajzen, I. and Fishbein, M. (1980), Understanding Attitudes and Predicting Social Behaviour, Prentice-Hall, Englewood Cliffs, NJ.
6) Psychological Bulletin, 82(2), 261. https://doi.org/10.1037/h0076477 Backfisch, I., Scherer, R., Siddiq, F., Lachner, A., & Scheiter, K. (2021). Teachers’ technology use for teaching: Comparing two explanatory mechanisms. Teaching and
7) Teacher Education, 104, 103390. https://doi.org/10.1016/j.tate.2021.103390 Bagozzi, R. P. (2007). The legacy of the technology acceptance model and a proposal for a paradigm shift. Journal of the Association for Information Systems, 8(4), 3.
8) Bandura, A. (1977). Self-efficacy: Toward a unifying theory of behavioral change.
9) Psychological Review, 84(2), 191. https://doi.org/10.1037/0033-295X.84.2.191 Bandura, A. (1986). The explanatory and predictive scope of self-efficacy theory. Journal of Clinical and Social Psychology, 4, 359–373. https://doi.org/10.1521/jscp.1986.4.3.359
10) Bagozzi (2007), “The legacy of technology acceptance model and a proposal for paradigm shift”,
11) Journal of the Association for Information Systems, Vol. 8 No. 4, pp. 244-254.
12) Baturay, M. H., Go¨kçearslan, S¸ ., & Ke, F. (2017). The relationship among pre-service teachers’ computer competence, attitude towards computer-assisted education, and intention of technology acceptance. International Journal of Technology Enhanced Learning, 9(1), 1–13. https://doi.org/10.1504/IJTEL.2017.084084
13) Benbasat, I., & Barki, H. (2007). Quo vadis TAM? Journal of the Association for Information Systems, 8(4), 7.
14) Bingimlas, K. A. (2009). Barriers to the successful integration of ICT in teaching and learning environments: A review of the literature. Eurasia Journal of Mathematics, Science and Technology Education, 5(3), 235–245. https://doi.org/10.12973/ejmste/ 75275
15) Black, R. A., Yang, Y., Beitra, D., & McCaffrey, S. (2015). Comparing fit and reliability estimates of a psychological instrument using second-order CFA, bifactor, and essentially tau-equivalent (coefficient alpha) models via AMOS 22. Journal of Psychoeducational Assessment, 33(5), 451–472. https://doi.org/10.1177/0734282914553551
16) Bollen, K. A., & Pearl, J. (2013). Eight myths about causality and structural equation models. In Handbook of causal analysis for social research (pp. 301–328). Dordrecht: Springer.
17) Bonoli, L., Berger, J.-L., & Lamamra, N. (Eds.). (2018). Enjeux de la formation professionnelle en Suisse. Le « mod`ele » suisse sous la loupe [Challenges for VET in Switzerland. The Swiss "model" under the microscope]. Seismo.
18) Brantley-Dias, L., & Ertmer, P. A. (2013). Goldilocks and TPACK: Is the construct ‘just right? Journal of Research on Technology in Education, 46(2), 103–128.
19) Byrne, B. M. (2016). Structural equation modeling with AMOS: Basic concepts, applications, and programming (3rd ed.). Routledge. https://doi.org/10.4324/9781315757421
20) Cattaneo, A., & Aprea, C. (2018). Visual technologies to bridge the gap between school and workplace in vocational education. In D. Ifenthaler (Ed.), Digital workplace learning. Bridging formal and informal learning with digital technologies (pp. 251–270). Springer. https://doi.org/10.1007/978-3-319-46215-8_14.
21) Cattaneo, A., Gurtner, J.-L., & Felder, J. (2022). Digital tools as boundary objects to support connectivity in dual vocational education: Towards a definition of design principles. In I. Zitter, E. Kyndt, & S. Beausaert (Eds.), At the intersection of (continuous) education and work: Practices and underlying principles (pp. 137–157). Routledge.
22) Chan, S.C. and Lu, M.T. (2004), “Understanding internet banking adoption and use behavior: a Hong Kong perspective”, Journal of Global Information Management, Vol. 12 No. 3, pp. 21-43.
23) Chen, R. (2010), “Investigating models of preservice teachers’ use of technology to support student-centered learning”, Computers & Education, Vol. 55 No. 1, pp. 32-42.
24) Chin, W.W. (1998), “The partial least squares approach to structural equation modeling”, Modern Methods for Business Research, Vol. 295 No. 2, pp. 295-336.
25) Cohen, J. (1988), Statistical Power Analysis for the Behavioral Sciences, Lawrence Erlbaum, Mahwah, NJ.
26) Compeau, D.R. and Higgins, C.A. (1995), “Computer self-efficacy: development of measure and initial test”, MIS Quarterly, Vol. 19 No. 2, pp. 189-211.
27) Davis, F. D., & Venkatesh, V. (1996). A critical assessment of potential measurement biases in the technology acceptance model: three experiments. International journal of human-computer studies, 45(1), 19–45. https://doi.org/10.1006/ijhc.1996.0040
28) Davis, F. D. (1989). Perceived usefulness, perceived ease of use, and user acceptance of information technology. MIS Quarterly, 13(3), 319–340. https://doi.org/10.2307/ 249008
29) Davis, F. D., Bagozzi, R. P., & Warshaw, P. R. (1989). User acceptance of computer technology: A comparison of two theoretical models. Management Science, 35(8), 982–1003. https://doi.org/10.1287/mnsc.35.8.982
30) Drossel, K., & Eickelmann, B. (2017). Teachers’ participation in professional development concerning the implementation of new technologies in class: A latent class analysis of teachers and the relationship with the use of computers, ICT self- efficacy and emphasis on teaching ICT skills. Large-scale Assessments in Education, 5 (1), 1–13. https://doi.org/10.1186/s40536-017-0053-7
31) Davis, F.D. (1986), “A technology acceptance model for empirically testing new end-user information systems: theory and results”, doctoral dissertation, Sloan School of Management, Massachusetts Institute of Technology, Cambridge, MA.
32) Davis, F.D. (1989), “Perceived usefulness, perceived ease of use, and user acceptance of information technology”, MIS Quarterly, Vol. 13 No. 3, pp. 319-340.
33) Davis, F.D., Bagozzi, R.P. and Warshaw, P.R. (1989), “User acceptance of computer technology: a comparison of two theoretical models”, Management Science, Vol. 35 No. 8, pp. 982-1003. JRIT&L 11,2 188
34) Dishaw, M.T. and Strong, D.M. (1999), “EXtending the technology acceptance model with task-technology fit constructs”, Information and Management, Vol. 36 No. 1, pp. 9-21.
35) Drennan, J., Kennedy, J. and Pisarksi, A. (2005), “Factors affecting student attitudes toward flexible online learning in management education”, The Journal of Educational Research, Vol. 9 No. 6, pp. 331-338.
36) Fishbein, M. and Ajzen, I. (1975), Belief, Attitude, Intention, and Behavior: An Introduction to Theory and Research, Addison-Wesley, Reading, MA.
37) Fraillon, J., Ainley, J., Schulz, W., Duckworth, D., & Friedman, T. (2019). IEA international computer and information literacy study 2018 assessment framework. Springer Nature. https://doi.org/10.1007/978-3-030-19389-8
38) From, J. (2017). Pedagogical digital competence—between values, knowledge and skills Higher Education Studies, 7(2), 43–50.
39) Fornell, C. and Larcker, D. (1981), “Evaluating structural equation models with unobservable variables and measurement error”, Journal of Marketing Research, Vol. 18 No. 1, pp. 39-50.
40) Garson, G. D. (2010). Structural equation modeling example using WinAMOS: The Wheaton study.
41) Ghomi, M., & Redecker, C. (2019). Digital competence of educators (DigCompEdu): Development and evaluation of a self-assessment instrument for teachers’ digital competence. CSEDU, 1, 541–548. https://doi.org/10.5220/0007679005410548.
42) Gil-Flores, J., Rodríguez-Santero, J., & Torres-Gordillo, J.-J. (2017). Factors that explain the use of ICT in secondary-education classrooms: The role of teacher characteristics and school infrastructure. Computers in Human Behavior, 68, 441–449. https://doi. org/10.1016/j.chb.2016.11.057
43) Grollmann, P. (2008). The quality of vocational teachers: Teacher education, institutional roles and professional reality. European Educational Research Journal, 7 (4), 535–547. https://doi.org/10.2304/eerj.2008.7.4.535
44) Gu`ardia, L., Maina, M., & Julia`, A. (2017). Digital competence assessment system:
45) Supporting teachers with the CRISS platform. In Central European conference on information and intelligent systems (pp. 77–82). Faculty of Organization and Informatics Varazdin.
46) Ha¨m¨ala¨inen, R., Nissinen, K., Mannonen, J., L¨amsa¨, J., Leino, K., & Taajamo, M. (2021). Understanding teaching professionals’ digital competence: What do PIAAC and TALIS reveal about technology-related skills, attitudes, and knowledge? Computers in
47) Hair, J.F., Hult, G.T.M., Ringle, C.M. and Sarstedt, M. (2014), A Primer on Partial Least Squares Structural Equation Modeling (pls–sem), Sage Publications, London.
48) Hair, J.F., Black, W.C., Babin, B.J., Anderson, R.E. and Tatham, R.L. (2006), Multivariate Data Analysis, 6th ed., Prentice-Hall International, Upper Saddle River, NJ.
49) Hair, J.F., Black, W.C., Babin, B.J., Anderson, R.E. and Tatham, R.L. (2010), Multivariate Data Analysis: A Global Perspective, Prentice-Hall International, NJ.
50) Hu, L.T. and Bentler, P.M. (1999), “Cutoff criteria for fit indexes in covariance structure analysis: ‘conventional criteria versus new alternatives. structural equation modeling”, A Multidisciplinary Journal, Vol. 6 No. 1, pp. 1-55.
51) Human Behavior, 117, 106672. https://doi.org/10.1016/j.chb.2020.106672 International Computer and Information Literacy Study. (2018). International association for the evaluation of educational achievement. ICILS. IEA https://www.iea.nl/studies/ iea/icils.
52) Holden and Rada (2011), “Understanding the influence of perceived usability and technology self-efficacy on teachers’ technology acceptance”, Journal of Research on Technology Education, Vol. 43 No. 4, pp. 343-367.
53) Hoyle, R.H. (2011), “Understanding the influence of perceived usability and technology self-efficacy on teachers’ technology acceptance”, Journal of Research on Technology Education, Vol. 43 No. 4, pp. 343-367.
54) Kline, R. B. (2016). Principles and practice of structural equation modeling. Guilford Press. Krumsvik, R. (2009). Situated learning in the network society and the digitised school. European Journal of Teacher Education, 32(2), 167–185. https://doi.org/10.1080/02619760802457224
55) King, W.R. and He, J. (2006), “A meta-analysis of the technology acceptance model”, Information & Management, Vol. 43 No. 6, pp. 740-755.
56) Krumsvik, R. J., Jones, L.Ø., Øfstegaard, M., & Eikeland, O. J. (2016). Upper secondary school teachers’ digital competence: Analysed by demographic, personal and professional characteristics. Nordic Journal of Digital Literacy, 11, 143–164, 03.
57) Kyndt, E., Beausaert, S., & Zitter, I. (Eds.). (2022). Developing connectivity between education and work: Principles and practices. Routledge. https://doi.org/10.4324/ 9781003091219.
58) Levin, B. B. (2014). The development of teachers’ beliefs. In International handbook of research on teachers’ beliefs (pp. 60–77). Routledge.
59) Lee, Y.-H., Hsieh, Y.-C. and Chen, Y.-H. (2013), “An investigation of employees’ use of e learning systems: applying the technology acceptance model”, Behaviour and Information Technology, Vol. 32 No. 2, pp. 173-189.
60) Legris, P., Ingham, J. and Collerette, P. (2003), “Why do people use information technology? A critical review of the technology acceptance model”, Information and Management, Vol. 40 No. 3, pp. 1-14.
61) Lucas, M., Bem-Haja, P., Siddiq, F., Moreira, A., & Redecker, C. (2021). The relation between in-service teachers’ digital competence and personal and contextual factors: What matters most? Computers & Education, 160, 104052. https://doi.org/10.1016/j.compedu.2020.104052
62) Luan, W.S., Fung, N.S., Nawawi, M. and Hong, T.S. (2005), “EXperienced and inexperienced internet users among pre-service teachers: their use and attitudes toward the internet”, Educational Technology & Society, Vol. 8 No. 1, pp. 90-103.
63) Maranguni´c, N., & Grani´c, A. (2015). Technology acceptance model: A literature review from 1986 to 2013. Universal Access in the Information Society, 14(1), 81–95. https:// doi.org/10.1007/s10209-014-0348-1
64) Nistor, N. (2014). When technology acceptance models won’t work: Non-significant intention-behavior effects. Computers in Human Behavior, 34, 299–300. https://doi. org/10.1016/j.chb.2014.02.052
65) Nunnally, J.C. and Bernstein, I.H. (1994), Psychometric Theory, McGraw-Hill, New York, NY.
66) Nunnally, J. C. (1978). Psychometric theory (2nd ed.). McGraw-Hill.
67) OECD. (2019). TALIS 2018 results (volume I): Teachers and school leaders as Lifelong learners. OECD Publishing. https://doi.org/10.1787/1d0bc92a-en
68) Park, S.Y. (2009), “An Analysis of the technology acceptance model in understanding university students’ behavioral intention to use e-learning”, Educational Technology & Society, Vol. 12 No. 3, pp. 150-162.
69) Pynoo, B., Devolder, P., Tondeur, J., van Braak, J., Duyck, W. and Duyck, P. (2011), “Predicting secondary school teachers’ acceptance and use of a digital learning environment: a cross-sectional study”, Computers in Human Behaviour, Vol. 27 No. 1, pp. 568-575.
70) Redecker, C. (2017). European framework for the digital competence of educators: DigCompEdu. In Y. Punie (Ed.), EUR 28775 EN. Publications Office of the European Union. https://doi.org/10.2760/159770.
71) Rienties, B., Giesbers, B., Lygo-Baker, S., Ma, H.W.S. and Rees, R. (2016), “Why some teachers easily learn to use a new virtual learning environment: a technology acceptance perspective”, Interactive Learning Environment, Vol. 24 No. 3, pp. 539-552.
72) Reisog˘lu, I., & Çebi, A. (2020). How can the digital competences of pre-service teachers be developed? EXamining a case study through the lens of DigComp and DigCompEdu. Computers & Education, 156, 103940. https://doi.org/10.1016/j. compedu.2020.103940
73) Sailer, M., Schultz-Pernice, F., & Fischer, F. (2021). Contextual facilitators for learning activities involving technology in higher education: The C♭-model. Computers in Human Behavior, 121, 106794. https://doi.org/10.1016/j.chb.2021.106794
74) S´anchez-Prieto, J. C., Olmos-Miguela´n˜ez, S., & García-Pen˜alvo, F. J. (2017). Mlearning and pre-service teachers: An assessment of the behavioral intention using an expanded TAM model. Computers in Human Behavior, 72, 644–654. https://doi.org/ 10.1016/j.chb.2016.09.061
75) Schaap, H., Baartman, L. K. J., & De Bruijn, E. (2012). Students’ learning processes during school-based learning and workplace learning in vocational education: A review. Vocations and Learning, 5, 99–117.
76) Scherer, R., Howard, S. K., Tondeur, J., & Siddiq, F. (2021). Profiling teachers’ readiness for online teaching and learning in higher education: Who’s ready? Computers in Human Behavior, 118, 106675. https://doi.org/10.1016/j.chb.2020.106675
77) Scherer, R., Siddiq, F., & Tondeur, J. (2019). The technology acceptance model (TAM): A meta-analytic structural equation modeling approach to explaining teachers’ adoption of digital technology in education. Computers & Education, 128, 13–35. https://doi.org/10.1016/j.compedu.2018.09.009
78) Scherer, R., & Teo, T. (2019). Unpacking teachers’ intentions to integrate technology: A meta-analysis. Educational Research Review, 27, 90–109. https://doi.org/10.1016/j. edurev.2019.03.001
79) Schumacker, R.E. and Lomax, R.G. (2010), A Beginner’s Guide to Structural Equation Modeling, Routledge, New York, NY.
80) Schreiber, J. B., Nora, A., Stage, F. K., Barlow, E. A., & King, J. (2006). Reporting structural equation modeling and confirmatory factor analysis results: A review. The Journal of Educational Research, 99(6), 323–338. https://doi.org/10.3200/ JOER.99.6.323-338
81) Schwendimann, B. A., Cattaneo, A. A., Dehler Zufferey, J., Gurtner, J. L., B´etrancourt, M., & Dillenbourg, P. (2015). The ‘Erfahrraum’: A pedagogical model for designing educational technologies in dual vocational systems. Journal of Vocational Education and Training, 67(3), 367–396. https://doi.org/10.1080/13636820.2015.1061041
82) Sime, D. and Priestley, M. (2003), “Student teachers’ first reflections on ICT in classroom learning: implications for initial teacher education”, Journal of Computer Assisted Learning, Vol. 21 No. 2, pp. 130-143.
83) Strahm, R. H., Geiger, B. H., Oertle, C., & Swars, E. (Eds.). (2016). Vocational and Professional Education and Training in Switzerland. Success factors and challenges for sustainable implementation abroad. hep Verlag.
84) Swanson, E.B. (1988), “Management information system: appreciation and involvement”, Management Science, Vol. 21 No. 2, pp. 178-188.
85) Tan, G.W.H., Sim, J.J., OOi, K.B. and Phusavat, K. (2012), “Determinants of mobile learning adoption: an empirical analysis”, Journal of Computer Information Systems, Vol. 52 No. 3, pp. 82-91.
86) Tondeur, J., Hermans, R., van Braak, J., & Valcke, M. (2008). EXploring the link between teachers’ educational belief profiles and different types of computer use in the classroom. Computers in Human Behavior, 24(6), 2541–2553. https://doi.org/10.1016/j.compedu.2007.05.003
87) Teo, T. (2010), “A path analysis of pre-service teachers’ attitudes to computer use: applying and extending the technology acceptance model in educational context”, Interactive Learning Environments, Vol. 18 No. 1, pp. 65-79.
88) Teo, T. (2012a), “EXamining the intention to use technology among pre-service teachers: an integration of the technology acceptance model and theory of planned behavior”, Interactive Learning Environments, Vol. 20 No. 1, pp. 3-18.
89) Teo, T. and van Schaik, P. (2012b), “Understanding the intention to use technology by preservice teachers: an empirical test of competing theoretical models”, International Journal of Human-Computer Interaction, Vol. 28 No. 3, pp. 178-188.
90) Thompson, R.L., Higgins, C.A. and Howell, J.M. (1991), “Personal computing: toward a conceptual model of utilization”, MIS Quarterly, Vol. 15 No. 1, pp. 124-143.
91) Taylor, S. and Todd, P.A. (1995), “Understanding information technology usage: a test of competing models”, Information Systems Research, Vol. 6 No. 2, pp. 144-176.
92) UNESCO. International Centre for Technical and Vocational Education and Training (UNESCO-UNEVOC). (2020). Promoting quality in TVET using technology: A practical guide.
93) Valtonen, T., Kukkonen, J., Kontkanen, S., Sormunen, K., Dillon, P., & Sointu, E. (2015). The impact of authentic learning experiences with ICT on pre-service teachers’ intentions to use ICT for teaching and learning. Computers & Education, 81, 49–58. https://doi.org/10.1016/j.compedu.2014.09.008
94) Venkatesh, V., & Bala, H. (2008). Technology acceptance model 3 and a research agenda on interventions. Decision Science, 39(2), 273–312.
95) Venkatesh, V., & Davis, F. D. (1996). A model of the antecedents of perceived ease of use: Development and test. Decision Sciences, 27(3), 451–481.
96) Venkatesh, V., & Davis, F. D. (2000). A theoretical extension of the technology acceptance model: Four longitudinal field studies. Management Science, 46(2), 186–204. https://doi.org/10.1287/mnsc.46.2.186.11926
97) Venkatesh, V., Morris, M. G., Davis, G. B., & Davis, F. D. (2003). User acceptance of information technology: Toward a unified view. MIS Quarterly, 425–478. https:// doi.org/10.2307/30036540
98) Wang, Y.-S., Wu, M.-C. and Wang, H.-Y. (2009), “Investigating the determinants and age and gender differences in the acceptance of mobile learning”, British Journal of Educational Technology, Vol. 40 No. 1, pp. 92-118.
99) Wong, K. T., Teo, T., & Russo, S. (2012). Influence of gender and computer teaching efficacy on computer acceptance among Malaysian student teachers: An extended technology acceptance model. Australasian Journal of Educational Technology, 28(7). https://doi.org/10.14742/ajet.796
100) Yuen, A.H.K. and Ma, W.W.K. (2008), “EXploring teacher acceptance of e-learning”, Asia Pacific Journal of Teacher Education, Vol. 36 No. 3, pp. 229-243.
101) Zarafshani, K., Solaymani, A., D’Itri, M., Helms, M. M., & Sanjabi, S. (2020). Evaluating technology acceptance in agricultural education in Iran: A study of vocational agriculture teachers. Social Sciences & Humanities Open, 2(1), 100041. https://doi. org/10.1016/j.ssaho.2020.100041
102) Zhao, Y., Llorente, A. M. P., & Go´mez, M. C. S. (2021). Digital competence in higher education research: A systematic literature review. Computers & Education, 104212. https://doi.org/10.1016/j.compedu.2021.104212.
Volume 07 Issue 11 November 2024
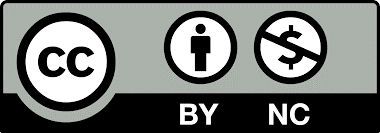
There is an Open Access article, distributed under the term of the Creative Commons Attribution – Non Commercial 4.0 International (CC BY-NC 4.0) (https://creativecommons.org/licenses/by-nc/4.0/), which permits remixing, adapting and building upon the work for non-commercial use, provided the original work is properly cited.
Our Services and Policies
Authors should prepare their manuscripts according to the instructions given in the authors' guidelines. Manuscripts which do not conform to the format and style of the Journal may be returned to the authors for revision or rejected.
The Journal reserves the right to make any further formal changes and language corrections necessary in a manuscript accepted for publication so that it conforms to the formatting requirements of the Journal.
International Journal of Multidisciplinary Research and Analysis will publish 12 monthly online issues per year,IJMRA publishes articles as soon as the final copy-edited version is approved. IJMRA publishes articles and review papers of all subjects area.
Open access is a mechanism by which research outputs are distributed online, Hybrid open access journals, contain a mixture of open access articles and closed access articles.
International Journal of Multidisciplinary Research and Analysis initiate a call for research paper for Volume 08 Issue 04 (April 2025).
PUBLICATION DATES:
1) Last Date of Submission : 26 April 2025.
2) Article published within a week.
3) Submit Article : editor@ijmra.in or Online
Why with us
1 : IJMRA only accepts original and high quality research and technical papers.
2 : Paper will publish immediately in current issue after registration.
3 : Authors can download their full papers at any time with digital certificate.
The Editors reserve the right to reject papers without sending them out for review.
Authors should prepare their manuscripts according to the instructions given in the authors' guidelines. Manuscripts which do not conform to the format and style of the Journal may be returned to the authors for revision or rejected. The Journal reserves the right to make any further formal changes and language corrections necessary in a manuscript accepted for publication so that it conforms to the formatting requirements of the Journal.