Mahdi Fadil Khaleel
Department of Community Health Techniques/Medical Technical Institute – Kirkuk, Northern Technical University, Iraq
DOI : https://doi.org/10.47191/ijmra/v8-i04-01Google Scholar Download Pdf
ABSTRACT:
Water scarcity has become of Iraq’s top and most serious challenges stemming from climate change, population increase and crumbling infrastructure. Sustainable development, in this context, can only be possible if new solutions are provided and among them recently Artificial Intelligence–AI, is one of the promising tools to deal with various water-related issues. This paper addresses the significance of artificial intelligence (AI) in solving the water challenges facing Iraq and scaling efforts towards achieving Sustainable Development Goal (SDG) number 6 on clean water and sanitation. Artificial intelligence technologies are used to store and analyze large datasets in order to optimize water resource management, improve irrigation, predict droughts, and track leaks in water distribution systems, such as machine learning, predictive analytics and remote sensing. So artificial intelligence can help the country allocate its water supply more efficiently and reduce waste, as it adapts to climate variability. Moreover, AI-based decision making can support decision makers in creating evidence-based strategies for sustainable water utilization. AI Is Poised to Change Iraq's Water Sector — But Data and Tech Infrastructures Lag Behind(https://civlified.com/ai-poised-change-iraq-water-sector-data-tech-infrastructures-lag-behind) The research reveals that despite AI technologies remain in their infancy, they can catalyze they are a catalyst for sustainable water management in Iraq and signify significant investments in information and communications technologies (ICT), capacity building and inter-sectoral collaboration are needed to realise their potential. So, by having AI in water management system, Iraq has one step closer to touch a sustainable development and have a vision of water security.
KEYWORDS:Sustainable Development, Clean Environment, Water Conservation, Artificial Intelligence, Water Protection in Iraq.
REFERENCES1) D.Pezzaniti Sharma, B. Myers, S. Cook, G. Tjandraatmadja, P. Chacko, S. Chavoshi,D. Kemp, R. Leonard, B. Koth, A. Walton, Water Sensitive Urban design: aninvestigation of current systems, implementation drivers, community perceptions and potential to supplement urban Water services, Water 8 (2016) 272, https://doi.org/10.3390/w8070272.
2) N. Doorn, Artificial intelligence in the water domain: opportunities for responsibleuse, Sci. Total Environ. 755 (2021) 142561, https://doi.org/10.1016/j.scitotenv.2020.142561.
3) C.E. Richards, A. Tzachor, S. Avin, R. Fenner, Rewards, risks and responsible deployment of artificial intelligence in water systems, Nat Water 1 (2023)422–432, https://doi.org/10.1038/s44221-023-00069-6.
4) C. Muldoon, L. Gorgü, ¨ J.J. O’Sullivan, W.G. Meijer, G.M.P. O’Hare, Miningexplainable predictive features for water quality management, (2022). https://doi.org/10.48550/ARXIV.2212.04419.
5) S. Koley, Sustainability appraisal of arsenic mitigation policy innovations in WestBengal, India. Infrastruc. Asset Manag 10 (1) (2022) 17–37, https://doi.org/10.1680/jinam.21.00021.
6) S.K. Roy, M.M. Hasan, I. Mondal, J. Akhter, S.K. Roy, S. Talukder, A.S. Islam,A. Rahman, S. Karuppannan, Empowered machine learning algorithm to identifysustainable groundwater potential zone map in Jashore District, Bangladesh.Groundw. Sustain. Dev. 25 (2024) 101168, https://doi.org/10.1016/j.gsd.2024.101168.
7) Z. Zong, Y. Guan, AI-driven intelligent data analytics and predictive analysis in industry 4.0: transforming knowledge, innovation, and efficiency, J. Knowl. Econ(2024) 1–40, https://doi.org/10.1007/s13132-024-02001-z.
8) S.E. Bibri, J. Huang, S.K. Jagatheesaperumal, J. Krogstie, The synergistic interplayof artificial intelligence and digital twin in environmentally planning sustainablesmart cities: a comprehensive systematic review, Environ. Sci. Ecotechnol. (2024)100433, https://doi.org/10.1016/j.ese.2024.100433.
9) S. Sicari, A. Rizzardi, C. Cappiello, D. Miorandi, A. Coen-Porisini, Toward DataGovernance in the Internet of Things, New advances in the internet of things, 2018,pp. 59–74, https://doi.org/10.1007/978-3-319-58190-3_4.
10) H.R. Maier, F.R. Taghikhah, E. Nabavi, S. Razavi, H. Gupta, W. Wu, D.A. Radford,J. Huang, How much X is in XAI: responsible use of “explainable” artificialintelligence in hydrology and water resources, J. Hydrol. X (2024) 100185, https://doi.org/10.1016/j.hydroa.2024.100185.
11) A. Weller, Transparency: motivations and challenges, in: W. Samek, G. Montavon,A. Vedaldi, L.K. Hansen, K.-R. Müller (Eds.), Explainable AI: Interpreting, Explaining and Visualizing Deep Learning, Springer International Publishing, Cham, 2019, pp. 23–40, https://doi.org/10.1007/978-3-030-28954-6_2.
12) C. Steging, S. Renooij, B. Verheij, Discovering the rationale of decisions:experiments on aligning learning and reasoning, (2021). https://doi.org/10.48550/ARXIV.2105.06758.
13) M. Ghassemi, L. Oakden-Rayner, A.L. Beam, The false hope of current approachesto explainable artificial intelligence in health care, Lancet Digit. Health 3 (2021)e745–e750, https://doi.org/10.1016/S2589-7500(21)00208-9.
14) D. Chakraborty, H. Bas¸agao ˘ glu, ˘ L. Gutierrez, A. Mirchi, Explainable AI reveals new hydroclimatic insights for ecosystem-centric groundwater management, Environ.Res. Lett. 16 (2021) 114024, https://doi.org/10.1088/1748-9326/ac2fde.
15) T. Chiaburu, F. Biessmann, Interpretable Time Series Models for WastewaterModeling, Combined Sewer Overflows, 2024, https://doi.org/10.48550/ARXIV.2401.02465.
16) P. Agrawal, A. Sinha, S. Kumar, A. Agarwal, A. Banerjee, V.G.K. Villuri, C.S.R. Annavarapu, R. Dwivedi, V.V.R. Dera, J. Sinha, S. Pasupuleti, Exploring artificialintelligence techniques for groundwater quality assessment, Water 13 (2021) 172, https://doi.org/10.3390/w13091172.
17) S. Ashraf, M. Akram, C. Jana, L. Jin, D. Pamucar, Multi-criteria assessment ofclimate change due to greenhouse effect based on Sugeno Weber model underspherical fuzzy Z-numbers, Inf Sci (Ny) 666 (2024) 120428, https://doi.org/10.1016/j.ins.2024.120428.
18) J. Cole, S. Sharvelle, N. Grigg, G. Pivo, J. Haukaas, Collaborative, risk-informed,triple bottom line, multi-criteria decision analysis planning framework forIntegrated Urban water management, Water. (Basel) 10 (2018) 1722, https://doi.org/10.3390/w10121722.
19) J. Sperling, W. Sarni, Sustainable and Resilient Water and Energy Futures: FromNew Ethics and Choices to Urban Nexus Strategies, in: P. ThanjavurFig. 5. Potential AI Risks in Water Systems That Could Result in Progress Traps.S.S. Infant et al. Results in Engineering 25 (2025) 10434912
20) G.D. Pelegrina, L.T. Duarte, M. Grabisch, A k-additive Choquet integral-basedapproach to approximate the SHAP values for local interpretability in machinelearning, Artif. Intell. 325 (2023) 104014, https://doi.org/10.1016/j.artint.2023.104014.
21) Y.H. Hung, C.Y. Lee, BMB-LIME: LIME with modeling local nonlinearity anduncertainty in explainability, Knowl. Based. Syst. 294 (2024) 111732, https://doi.org/10.1016/j.knosys.2024.111732.
22) A. Inglis, Parnell A, C.B. Hurley, Visualizing variable importance and variableinteraction effects in machine learning models, J. Comput. Graph. Stat. 31 (3)(2022) 766–778, https://doi.org/10.1080/10618600.2021.2007935.
23) C. Maußner, M. Oberascher, A. Autengruber, A. Kahl, R. Sitzenfrei, Explainableartificial intelligence for reliable water demand forecasting to increase trust inpredictions, Water. Res. 268 (2025) 122779, https://doi.org/10.1016/j.watres.2024.122779.
24) F.-J. Chang, L.-C. Chang, J.-F. Chen, Artificial intelligence techniques in hydrologyand water resources management, Water. (Basel) 15 (2023) 1846, https://doi.org/10.3390/w15101846.
25) Y. Li, S. Mao, Y. Yuan, Z. Wang, Y. Kang, Y. Yao, Beyond tides and time: machinelearning triumph in water quality, (2023). https://doi.org/10.48550/ARXIV.2309.
26) F. Al Azzo et al., “Advanced methods for identifying counterfeit currency: Using deep learning and machine learning,” NTU Journal of Engineering and Technology 3 (2024).
27) M. Hameed and R. R. O. Al-Nima, “High-performance character recognition system utilizing deep convolutional neural networks,” NTU Journal of Engineering and Technology 3, 42–51 (2024)
Volume 08 Issue 04 April 2025
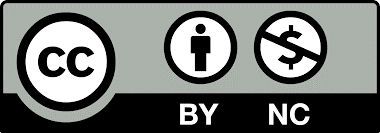
There is an Open Access article, distributed under the term of the Creative Commons Attribution – Non Commercial 4.0 International (CC BY-NC 4.0) (https://creativecommons.org/licenses/by-nc/4.0/), which permits remixing, adapting and building upon the work for non-commercial use, provided the original work is properly cited.
Our Services and Policies
Authors should prepare their manuscripts according to the instructions given in the authors' guidelines. Manuscripts which do not conform to the format and style of the Journal may be returned to the authors for revision or rejected.
The Journal reserves the right to make any further formal changes and language corrections necessary in a manuscript accepted for publication so that it conforms to the formatting requirements of the Journal.
International Journal of Multidisciplinary Research and Analysis will publish 12 monthly online issues per year,IJMRA publishes articles as soon as the final copy-edited version is approved. IJMRA publishes articles and review papers of all subjects area.
Open access is a mechanism by which research outputs are distributed online, Hybrid open access journals, contain a mixture of open access articles and closed access articles.
International Journal of Multidisciplinary Research and Analysis initiate a call for research paper for Volume 08 Issue 04 (April 2025).
PUBLICATION DATES:
1) Last Date of Submission : 26 April 2025.
2) Article published within a week.
3) Submit Article : editor@ijmra.in or Online
Why with us
1 : IJMRA only accepts original and high quality research and technical papers.
2 : Paper will publish immediately in current issue after registration.
3 : Authors can download their full papers at any time with digital certificate.
The Editors reserve the right to reject papers without sending them out for review.
Authors should prepare their manuscripts according to the instructions given in the authors' guidelines. Manuscripts which do not conform to the format and style of the Journal may be returned to the authors for revision or rejected. The Journal reserves the right to make any further formal changes and language corrections necessary in a manuscript accepted for publication so that it conforms to the formatting requirements of the Journal.